There’s a famous parable about a group of blind men encountering an elephant for the very first time. The first blind man, who had his hand on the elephant’s side, said that it was like an enormous wall. The second blind man, wrapping his arms around the elephant’s leg, exclaimed that surely it was a gigantic tree trunk. The third, feeling the elephant’s tail, declared that it must be a thick rope. Vehement disagreement ensues, but after a while the blind men eventually come to realize that, while each person was partially correct, there is much more to the elephant than initially thought.
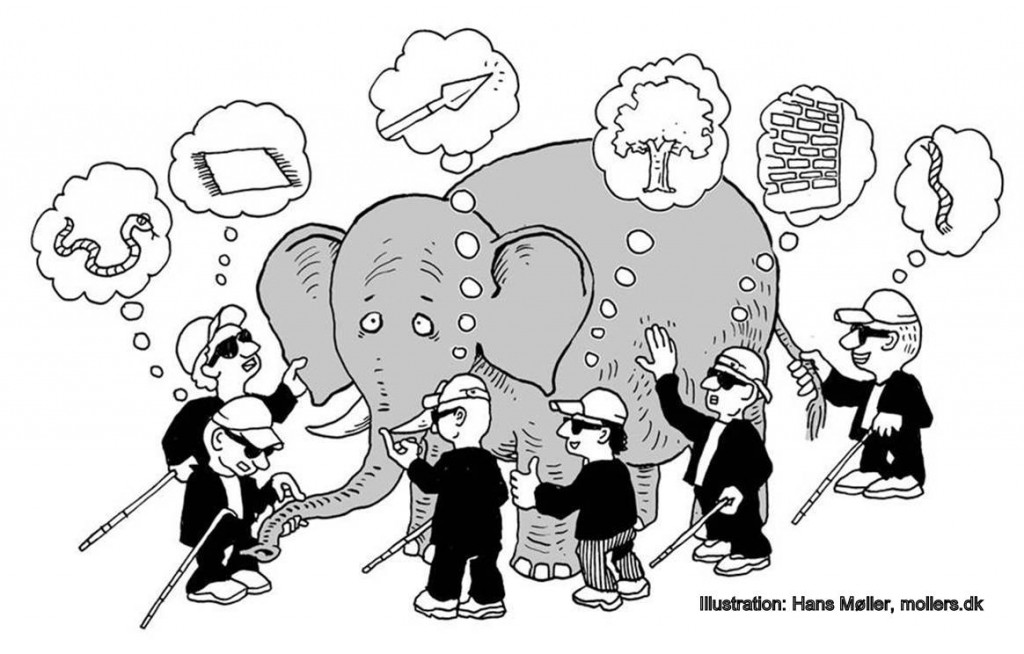
Last month, Zhengfeng, Anand, Thomas, John and I posted MIP* = RE to arXiv. The paper feels very much like the elephant of the fable — and not just because of the number of pages! To a computer scientist, the paper is ostensibly about the complexity of interactive proofs. To a quantum physicist, it is talking about mathematical models of quantum entanglement. To the mathematician, there is a claimed resolution to a long-standing problem in operator algebras. Like the blind men of the parable, each are feeling a small part of a new phenomenon. How do the wall, the tree trunk, and the rope all fit together?
I’ll try to trace the outline of the elephant: it starts with a mystery in quantum complexity theory, curves through the mathematical foundations of quantum mechanics, and arrives at a deep question about operator algebras.
The rope: The complexity of nonlocal games
In 2004, computer scientists Cleve, Hoyer, Toner, and Watrous were thinking about a funny thing called nonlocal games. A nonlocal game
involves three parties: two cooperating players named Alice and Bob, and someone called the verifier. The verifier samples a pair of random questions
and sends
to Alice (who responds with answer
), and
to Bob (who responds with answer
). The verifier then uses some function
that tells her whether the players win, based on their questions and answers.
All three parties know the rules of the game before it starts, and Alice and Bob’s goal is to maximize their probability of winning the game. The players aren’t allowed to communicate with each other during the game, so it’s a nontrivial task for them to coordinate an optimal strategy (i.e., how they should individually respond to the verifier’s questions) before the game starts.
The most famous example of a nonlocal game is the CHSH game (which has made several appearances on this blog already): in this game, the verifier sends a uniformly random bit
to Alice (who responds with a bit
) and a uniformly random bit
to Bob (who responds with a bit
). The players win if
(in other words, the sum of their answer bits is equal to the product of the input bits modulo
).
What is Alice’s and Bob’s maximum winning probability? Well, it depends on what type of strategy they use. If they use a strategy that can be modeled by classical physics, then their winning probability cannot exceed
(we call this the classical value of CHSH). On the other hand, if they use a strategy based on quantum physics, Alice and Bob can do better by sharing two quantum bits (qubits) that are entangled. During the game each player measures their own qubit (where the measurement depends on their received question) to obtain answers that win the CHSH game with probability
(we call this the quantum value of CHSH). So even though the entangled qubits don’t allow Alice and Bob to communicate with each other, entanglement gives them a way to win with higher probability! In technical terms, their responses are more correlated than what is possible classically.
The CHSH game comes from physics, and was originally formulated not as a game involving Alice and Bob, but rather as an experiment involving two spatially separated devices to test whether stronger-than-classical correlations exist in nature. These experiments are known as Bell tests, named after John Bell. In 1964, he proved that correlations from quantum entanglement cannot be explained by any “local hidden variable theory” — in other words, a classical theory of physics. He then showed that a Bell test, like the CHSH game, gives a simple statistical test for the presence of nonlocal correlations between separated systems. Since the 1960s, numerous Bell tests have been conducted experimentally, and the verdict is clear: nature does not behave classically.
Cleve, Hoyer, Toner and Watrous noticed that nonlocal games/Bell tests can be viewed as a kind of multiprover interactive proof. In complexity theory, interactive proofs are protocols where some provers are trying to convince a verifier of a solution to a long, difficult computation, and the verifier is trying to efficiently determine if the solution is correct. In a Bell test, one can think of the provers as instead trying to convince the verifier of a physical statement: that they possess quantum entanglement.
With the computational lens trained firmly on nonlocal games, it then becomes natural to ask about their complexity. Specifically, what is the complexity of approximating the optimal winning probability in a given nonlocal game
? In complexity-speak, this is phrased as a question about characterizing the class MIP* (pronounced “M-I-P star”). This is also a well-motivated question for an experimentalist conducting Bell tests: at the very least, they’d want to determine if (a) quantum players can do better than classical players, and (b) what can the best possible quantum strategy achieve?
Studying this question in the case of classical players led to some of the most important results in complexity theory, such as MIP = NEXP and the PCP Theorem. Indeed, the PCP Theorem says that it is NP-hard to approximate the classical value of a nonlocal game (i.e. the maximum winning probability of classical players) to within constant additive accuracy (say
). Thus, assuming that P is not equal to NP, we shouldn’t expect a polynomial-time algorithm for this. However it is easy to see that there is a “brute force” algorithm for this problem: by taking exponential time to enumerate over all possible deterministic player strategies, one can exactly compute the classical value of nonlocal games.
When considering games with entangled players, however, it’s not even clear if there’s a similar “brute force” algorithm that solves this in any amount of time — forget polynomial time; even if we allow ourselves exponential, doubly-exponential, Ackermann function amount of time, we still don’t know how to solve this quantum value approximation problem. The problem is that there is no known upper bound on the amount of entanglement that is needed for players to play a nonlocal game. For example, for a given game
, does an optimal quantum strategy require one qubit, ten qubits, or
qubits of entanglement? Without any upper bound, a “brute force” algorithm wouldn’t know how big of a quantum strategy to search for — it would keep enumerating over bigger and bigger strategies in hopes of finding a better one.
Thus approximating the quantum value may not even be solvable in principle! But could it really be uncomputable? Perhaps we just haven’t found the right mathematical tool to give an upper bound on the dimension — maybe we just need to come up with some clever variant of, say, Johnson-Lindenstrauss or some other dimension reduction technique.
In 2008, there was promising progress towards an algorithmic solution for this problem. Two papers [DLTW, NPA] (appearing on arXiv on the same day!) showed that an algorithm based on semidefinite programming can produce a sequence of numbers that converge to something called the commuting operator value of a nonlocal game. If one could show that the commuting operator value and the quantum value of a nonlocal game coincide, then this would yield an algorithm for solving this approximation problem!
Asking whether this commuting operator and quantum values are the same, however, immediately brings us to the precipice of some deep mysteries in mathematical physics and operator algebras, far removed from computer science and complexity theory. This takes us to the next part of the elephant.
The tree: mathematical foundations of locality
The mystery about the quantum value versus the commuting operator value of nonlocal games has to do with two different ways of modeling Alice and Bob in quantum mechanics. As I mentioned earlier, quantum physics predicts that the maximum winning probability in, say, the CHSH game when Alice and Bob share entanglement is approximately 85%. As with any physical theory, these predictions are made using some mathematical framework — formal rules for modeling physical experiments like the CHSH game.
In a typical quantum information theory textbook, players in the CHSH game are usually modelled in the following way: Alice’s device is described a state space
(all the possible states the device could be in), a particular state
from
, and a set of measurement operators
(operations that can be performed by the device). It’s not necessary to know what these things are formally; the important feature is that these three things are enough to make any prediction about Alice’s device — when treated in isolation, at least. Similarly, Bob’s device can be described using its own state space
, state
, and measurement operators
.
In the CHSH game though, one wants to make predictions about Alice’s and Bob’s devices together. Here the textbooks say that Alice and Bob are jointly described by the tensor product formalism, which is a natural mathematical way of “putting separate spaces together”. Their state space is denoted by
. The joint state
describing the devices comes from this tensor product space. When Alice and Bob independently make their local measurements, this is described by a measurement operator from the tensor product of operators from
and
. The strange correlations of quantum mechanics arise when their joint state
is entangled, i.e. it cannot be written as a well-defined state on Alice’s side combined with a well-defined state on Bob’s side (even though the state space itself is two independent spaces combined together!)
The tensor product model works well; it satisfies natural properties you’d want from the CHSH experiment, such as the constraint that Alice and Bob can’t instantaneously signal to each other. Furthermore, predictions made in this model match up very accurately with experimental results!
This is the not the whole story, though. The tensor product formalism works very well in non-relativistic quantum mechanics, where things move slowly and energies are low. To describe more extreme physical scenarios — like when particles are being smashed together at near-light speeds in the Large Hadron Collider — physicists turn to the more powerful quantum field theory. However, the notion of spatiotemporal separation in relativistic settings gets especially tricky. In particular, when trying to describe quantum mechanical systems, it is no longer evident how to assign Alice and Bob their own independent state spaces, and thus it’s not clear how to put relativistic Alice and Bob in the tensor product framework!
In quantum field theory, locality is instead described using the commuting operator model. Instead of assigning Alice and Bob their own individual state spaces and then tensoring them together to get a combined space, the commuting operator model stipulates that there is just a single monolithic space
for both Alice and Bob. Their joint state is described using a vector
from
, and Alice and Bob’s measurement operators both act on
. The constraint that they can’t communicate is captured by the fact that Alice’s measurement operators commute with Bob’s operators. In other words, the order in which the players perform their measurements on the system does not matter: Alice measuring before Bob, or Bob measuring before Alice, both yield the same statistical outcomes. Locality is enforced through commutativity.
The commuting operator framework contains the tensor product framework as a special case, so it’s more general. Could the commuting operator model allow for correlations that can’t be captured by the tensor product model, even approximately? This question is known as Tsirelson’s problem, named after the late mathematician Boris Tsirelson.
There is a simple but useful way to phrase this question using nonlocal games. What we call the “quantum value” of a nonlocal game
(denoted by
) really refers to the supremum of success probabilities over tensor product strategies for Alice and Bob. If they use strategies from the more general commuting operator model, then we call their maximum success probability the commuting operator value of
(denoted by
). Since tensor product strategies are a special case of commuting operator strategies, we have the relation
for all nonlocal games
.
Could there be a nonlocal game
whose tensor product value is different from its commuting operator value? With tongue-in-cheek: is there a game
that Alice and Bob could succeed at better if they were using quantum entanglement at near-light speeds? It is difficult to find even a plausible candidate game for which the quantum and commuting operator values may differ. The CHSH game, for example, has the same quantum and commuting operator value; this was proved by Tsirelson.
If the tensor product and the commuting operator models are the same (i.e., the “positive” resolution of Tsirelson’s problem), then as I mentioned earlier, this has unexpected ramifications: there would be an algorithm for approximating the quantum value of nonlocal games.
How does this algorithm work? It comes in two parts: a procedure to search from below, and one to search from above. The “search from below” algorithm computes a sequence of numbers
where
is (approximately) the best winning probability when Alice and Bob use a
-qubit tensor product strategy. For fixed
, the number
can be computed by enumerating over (a discretization of) the space of all possible
-qubit strategies. This takes a doubly-exponential amount of time in
— but at least this is still a finite time! This naive “brute force” algorithm will slowly plod along, computing a sequence of better and better winning probabilities. We’re guaranteed that in the limit as
goes to infinity, the sequence
converges to the quantum value
. Of course the issue is that the “search from below” procedure never knows how close it is to the true quantum value.
This is where the “search from above” comes in. This is an algorithm that computes a different sequence of numbers
where each
is an upper bound on the commuting operator value
, and furthermore as
goes to infinity,
eventually converges to
. Furthermore, each
can be computed by a technique known as semidefinite optimization; this was shown by the two papers I mentioned.
Let’s put the pieces together. If the quantum and commuting operator values of a game
coincide (i.e.
), then we can run the “search from below” and “search from above” procedures in parallel, interleaving the computation of the
and
. Since both are guaranteed to converge to the quantum value, at some point the upper bound
will come within some
to the lower bound
, and thus we would have homed in on (an approximation of)
. There we have it: an algorithm to approximate the quantum value of games.
All that remains to do, surely, is to solve Tsirelson’s problem in the affirmative (that commuting operator correlations can be approximated by tensor product correlations), and then we could put this pesky question about the quantum value to rest. Right?
The wall: Connes’ embedding problem
At the end of the 1920s, polymath extraordinaire John von Neumann formulated the first rigorous mathematical framework for the recently developed quantum mechanics. This framework, now familiar to physicists and quantum information theorists everywhere, posits that quantum states are vectors in a Hilbert space, and measurements are linear operators acting on those spaces. It didn’t take long for von Neumann to realize that there was a much deeper theory of operators on Hilbert spaces waiting to be discovered. With Francis Murray, in the 1930s he started to develop a theory of “rings of operators” — today these are called von Neumann algebras.
The theory of operator algebras has since flourished into a rich and beautiful area of mathematics. It remains inseparable from mathematical physics, but has established deep connections with subjects such as knot theory and group theory. One of the most important goals in operator algebras has been to provide a classification of von Neumann algebras. In their series of papers on the subject, Murray and von Neumann first showed that classifying von Neumann algebras reduces to understanding their factors, the atoms out of which all von Neumann algebras are built. Then, they showed that factors of von Neumann algebras come in one of three species: type
, type
, and type
. Type
factors were completely classified by Murray and von Neumann, and they made much progress on characterizing certain type
factors. However progress stalled until the 1970s, when Alain Connes provided a classification of type
factors (work for which he would later receive the Fields Medal). In the same 1976 classification paper, Connes makes a casual remark about something called type
factors:
We now construct an embedding of
into
. Apparently such an embedding ought to exist for all
factors.
This line, written in almost a throwaway manner, eventually came to be called “Connes’ embedding problem”: does every separable
factor embed into an ultrapower of the hyperfinite
factor? It seems that Connes surmises that it does (and thus this is also called “Connes’ embedding conjecture“). Since 1976, this problem has grown into a central question of operator algebras, with numerous equivalent formulations and consequences across mathematics.
In 2010, two papers (again appearing on the arXiv on the same day!) showed that the reach of Connes’ embedding conjecture extends back to the foundations of quantum mechanics. If Connes’ embedding problem has a positive answer (i.e. an embedding exists), then Tsirelson’s problem (i.e. whether commuting operator can be approximated by tensor product correlations) also has a positive answer! Later it was shown by Ozawa that Connes’ embedding problem is in fact equivalent to Tsirelson’s problem.
Remember that our approach to compute the value of nonlocal games hinged on obtaining a positive answer to Tsirelson’s problem. The sequence of papers [NPA, DLTW, Fritz, JNPPSW] together show that resolving — one way or another — whether this search-from-below, search-from-above algorithm works would essentially settle Connes’ embedding conjecture. What started as a funny question at the periphery of computer science and quantum information theory has morphed into an attack on one of the central problems in operator algebras.
MIP* = RE
We’ve now ended back where we started: the complexity of nonlocal games. Let’s take a step back and try to make sense of the elephant.
Even to a complexity theorist, “MIP* = RE” may appear esoteric. The complexity classes MIP* and RE refer to a bewildering grabbag of concepts: there’s Alice, Bob, Turing machines, verifiers, interactive proofs, quantum entanglement. What is the meaning of the equality of these two classes?
First, it says that the Halting problem has an interactive proof involving quantum entangled provers. In the Halting problem, you want to decide whether a Turing machine
, if you started running it, would eventually terminate with a well-defined answer, or if it would get stuck in an infinite loop. Alan Turing showed that this problem is undecidable: there is no algorithm that can solve this problem in general. Loosely speaking, the best thing you can do is to just flick on the power switch to
, and wait to see if it eventually stops. If
gets stuck in an infinite loop — well, you’re going to be waiting forever.
MIP* = RE shows with the help of all-powerful Alice and Bob, a time-limited verifier can run an interactive proof to “shortcut” the waiting. Given the Turing machine
‘s description (its “source code”), the verifier can efficiently compute a description of a nonlocal game
whose behavior reflects that of
. If
does eventually halt (which could happen after a million years), then there is a strategy for Alice and Bob that causes the verifier to accept with probability
. In other words,
. If
gets stuck in an infinite loop, then no matter what strategy Alice and Bob use, the verifier always rejects with high probability, so
is close to
.
By playing this nonlocal game, the verifier can obtain statistical evidence that
is a Turing machine that eventually terminates. If the verifier plays
and the provers win, then the verifier should believe that it is likely that
halts. If they lose, then the verifier concludes there isn’t enough evidence that
halts. The verifier never actually runs
in this game; she has offloaded the task to Alice and Bob, who we can assume are computational gods capable of performing million-year-long computations instantly. For them, the challenge is instead to convince the verifier that if she were to wait millions of years, she would witness the termination of
. Incredibly, the amount of work put in by the verifier in the interactive proof is independent of the time it takes for
to halt!
The fact that the Halting problem has an interactive proof seems borderline absurd: if the Halting problem is unsolvable, why should we expect it to be verifiable? Although complexity theory has taught us that there can be a large gap between the complexity of verification versus search, it has always been a difference of efficiency: if solutions to a problem can be efficiently verified, then solutions can also be found (albeit at drastically higher computational cost). MIP* = RE shows that, with quantum entanglement, there can be a chasm of computability between verifying solutions and finding them.
Now let’s turn to the non-complexity consequences of MIP* = RE. The fact that we can encode the Halting problem into nonlocal games also immediately tells us that there is no algorithm whatsoever to approximate the quantum value. Suppose there was an algorithm that could approximate
. Then, using the transformation from Turing machines to nonlocal games mentioned above, we could use this algorithm to solve the Halting problem, which is impossible.
Now the dominoes start to fall. This means that, in particular, the proposed “search-from-below”/”search-from-above” algorithm cannot succeed in approximating
. There must be a game
, then, for which the quantum value is different from the commuting operator value. But this implies Tsirelson’s problem has a negative answer, and therefore Connes’ embedding conjecture is false.
We’ve only sketched the barest of outlines of this elephant, and yet it is quite challenging to hold it in the mind’s eye all at once. This story is intertwined with some of the most fundamental developments in the past century: modern quantum mechanics, operator algebras, and computability theory were birthed in the 1930s. Einstein, Podolsky and Rosen wrote their landmark paper questioning the nature of quantum entanglement in 1935, and John Bell discovered his famous test and inequality in 1964. Connes’ formulated his conjecture in the ’70s, Tsirelson made his contributions to the foundations of quantum mechanics in the ’80s, and about the same time computer scientists were inventing the theory of interactive proofs and probabilistically checkable proofs (PCPs).
We haven’t said anything about the proof of MIP* = RE yet (this may be the subject of future blog posts), but it is undeniably a product of complexity theory. The language of interactive proofs and Turing machines is not just convenient but necessary: at its heart MIP* = RE is the classical PCP Theorem, with the help of quantum entanglement, recursed to infinity.
What is going on in this proof? What parts of it are fundamental, and which parts are unnecessary? What is the core of it that relates to Connes’ embedding conjecture? Are there other consequences of this uncomputability result? These are questions to be explored in the coming days and months, and the answers we find will be fascinating.
Acknowledgments. Thanks to William Slofstra and Thomas Vidick for helpful feedback on this post.